What is generative AI? How does it work?
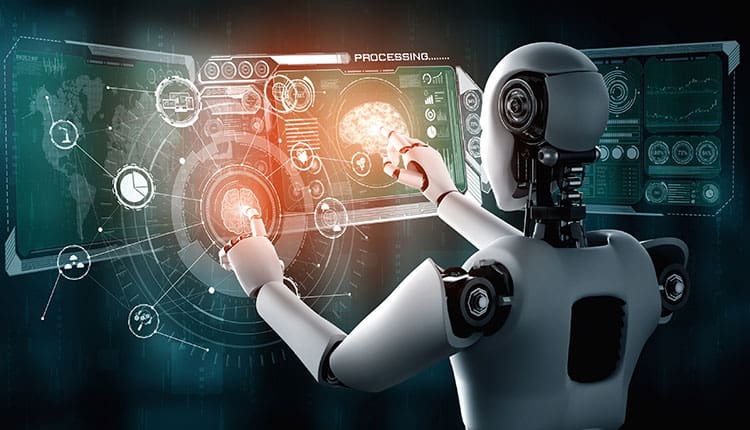
Introduction: Generative Artificial Intelligence (AI) represents a fascinating frontier in machine learning, enabling computers to create new content, such as images, text, and music, that closely resembles human-generated content. In this blog post, we will explore the concept of generative AI, its underlying mechanisms, and its diverse applications across various domains.
Understanding Generative AI: Generative AI refers to a subset of AI techniques that focus on generating new data samples that mimic the characteristics of a given dataset. Unlike discriminative models, which aim to classify or label data, generative models learn the underlying probability distribution of the data and generate new samples from this distribution.
How Generative AI Works: Generative AI techniques leverage various algorithms and architectures to learn the underlying patterns and structures present in the data and generate new samples that resemble the original data. Some common approaches to generative AI include:
- Generative Adversarial Networks (GANs): GANs consist of two neural networks—the generator and the discriminator—that are trained simultaneously in a competitive manner. The generator generates fake data samples, while the discriminator distinguishes between real and fake samples. Through iterative training, the generator learns to produce increasingly realistic samples that fool the discriminator.
- Variational Autoencoders (VAEs): VAEs are probabilistic generative models that learn to encode input data into a lower-dimensional latent space and then decode it back into the original data space. By sampling from the learned latent space, VAEs can generate new data samples that exhibit similar characteristics to the training data.
- Autoregressive Models: Autoregressive models generate data sequentially, one element at a time, based on the probability distribution of the data and the previously generated elements. Examples of autoregressive models include recurrent neural networks (RNNs) and transformers, which have been used for generating sequential data such as text and music.
Applications of Generative AI: Generative AI has diverse applications across various domains, including:
- Image Generation: Generative models such as GANs can generate realistic images of faces, landscapes, and objects.
- Text Generation: Language models like GPT-3 can generate coherent and contextually relevant text across a wide range of topics.
- Creative Design: Generative AI enables the creation of novel designs, artworks, and music compositions.
- Data Augmentation: Generative models can be used to augment training data for machine learning models, improving their performance and generalization.
Conclusion: In conclusion, generative AI represents a powerful paradigm that enables computers to create new content with remarkable realism and creativity. By harnessing advanced algorithms and architectures, generative AI holds the potential to revolutionize various industries and unlock new opportunities for innovation and expression.
Read More: What is the best diet and food for a healthy body?